Publications
Testing a Distributional Semantics Account of Grammatical Gender Effects on Semantic Gender Perception
George Flint, Anna Ivanova
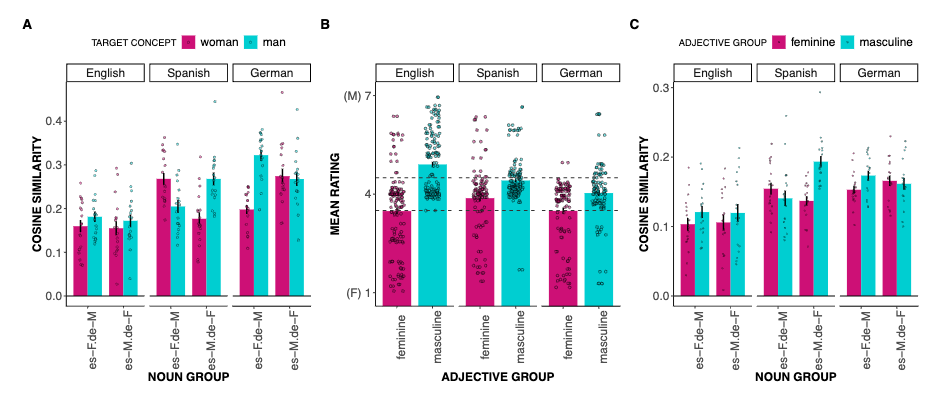
One well-known prediction of linguistic relativity theories is the effect of a noun’s grammatical gender on its semantics; for instance, ”key” is feminine in Spanish but masculine in German and thus might be associated with feminine traits for Spanish speakers but with masculine traits for German speakers. Experimental and corpus evidence for these effects has been mixed. In this work, we considered a distributional semantics account of putative grammatical gender effects on semantics and tested its predictions in Spanish, German, and English (control). In Part 1, we hypothesized that grammatical gender of concrete nouns affects the similarity of noun embeddings to embeddings of adjectives semantically associated with men or with women. We found support for this hypothesis in fastText embeddings, showing that nouns with the same meaning but with opposite genders in Spanish and German show opposite attraction effects both for words ”man” and ”woman” and for adjectives associated with men and women, although the effect size was weaker for German than for Spanish. BERT embeddings also showed consistent effects for Spanish but mixed results for German, suggesting possible variation across languages. In Part 2, we asked whether people systematically choose adjectives associated with women/men for grammatically feminine/masculine nouns, respectively. In a noun-adjective matching experiment (432 participants total), we found predicted grammatical gender effects for Spanish but not for German. Cosine similarity between the noun and the adjectives in fastText embeddings significantly predicted trial-level responses in all 3 languages; however, Spanish showed an additional effect of grammatical gender, indicating that participant noun-adjective associations are not fully explained by distributional semantics.
Language use is only sparsely compositional: The case of English adjective-nounphrases in humans and large language models
Aalok Sathe, George Flint, Evelina Fedorenko,* Noga Zaslavsky*
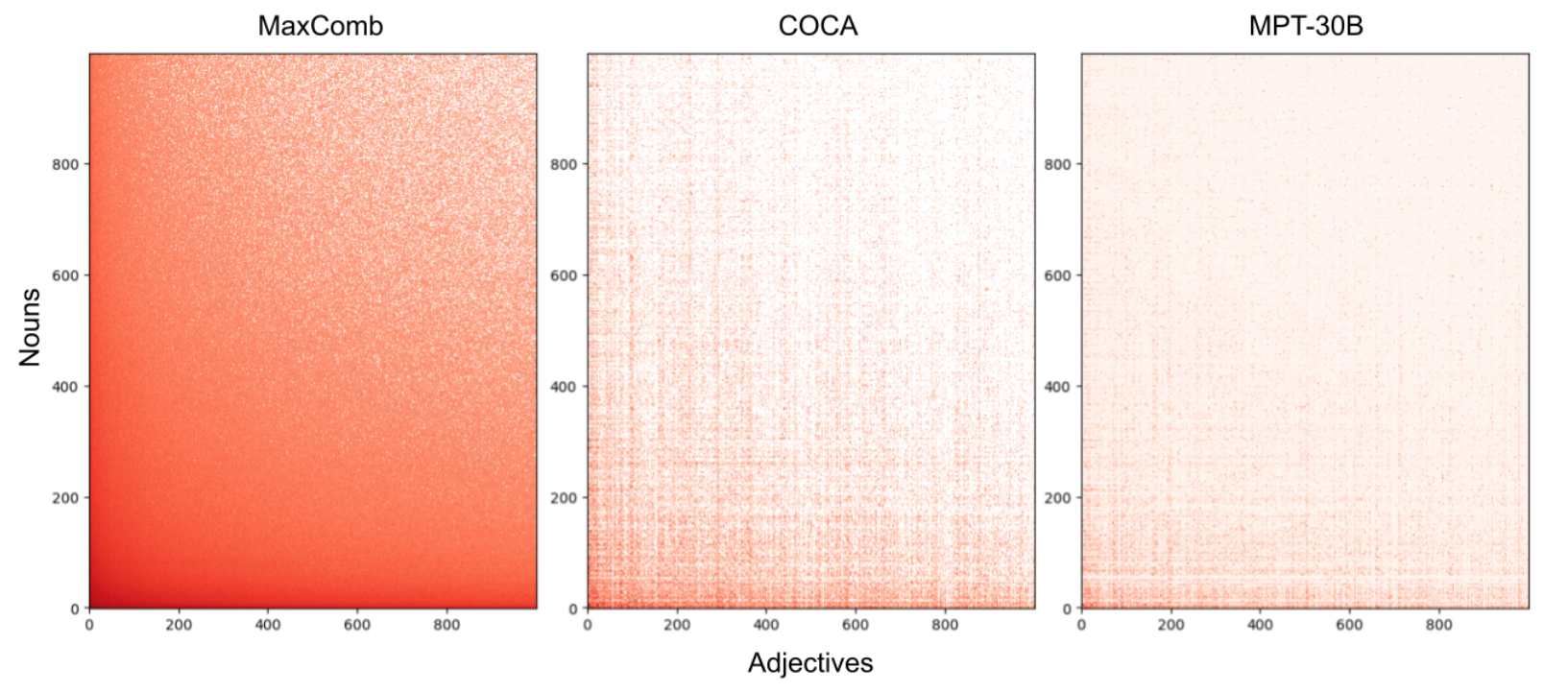
Compositionality is considered a key hallmark of human language. However, most research focuses on item-level compositionality, e.g., to what extent the meanings of phrases are composed of the meanings of their sub-parts, rather than on language-level compositionality, which is the degree to which possible combinations are utilized in practice during language use. Here, we propose a novel way to quantify the degree of language-level compositionality and apply it in the case of English adjective-noun combinations. Using corpus analyses, large language models, and human acceptability ratings, we find that (1) English only sparsely utilizes the compositional potential of adjective-noun combinations; and (2) LLMs struggle to predict human acceptability judgments of rare combinations. Taken together, our findings shed new light on the role of compositionality in language and highlight a challenging area for further improving LLMs.
Testing Evolutionary and Reinforcement Learning Approaches to Traffic Flow Optimization in SUMO
Dominic Domingo, Aryan Bandi, Arya Kunisetty, Ahan Banerjee, George Flint*, Kevin Zhu*
%2016.55.34.png)
Urban road systems require intricate planning to ensure safe and efficient transportation. Effective road systems help reduce traffic congestion, maximize throughput, and minimize collisions. Previous applications of machine learning algorithms to this topic have largely been focused on prediction, not optimization. In this work, we train and evaluate evolutionary and reinforcement learning models on this optimization problem by interfacing with the Simulation of Urban Mobility (SUMO) package. SUMO offers a framework to model and evaluate traffic dynamics, allowing users to configure parameters to explore the effect of various configurations on evaluation metrics. Models set values for traffic signal timings, speed limits, and designated lane access across a road network. They are evaluated on metrics for vehicle throughput, total waiting time, total travel time, speed variation, and crash frequency. We observe performance improvements compared to configurations that estimate the corresponding real-world networks, indicating evolutionary and reinforcement learning approaches might be well suited for this task, despite sparse application thus far.
AAAI Workshop on AI for Urban Planning
preprint* = senior author

Copyright © 2025 George Flint - All rights reserved.